Now available: Hardware accelerated, unsupervised, codec modernization to AV1 for increased efficiency video AI workflows
AV1, the new kid on the block of video encoders, is definitely starting to gain traction due to its high compression efficiency and increasing adoption on browsers and end devices. As we mentioned in our previous blog, H/W accelerated AV1 encoding is a particularly attractive prospect due to the combination of increased efficiency and light speed performance. H/W accelerated codec modernization – using Beamr’s Content Adaptive Bit-Rate (CABR) video optimization process running with NVIDIA video encoding – allows for fast, fully automatic, upgrade of legacy encodes to perceptually identical AV1 encodes.
Codec modernization is essentially the ability to get double the benefit – both the increased compression efficiency of codecs such as AV1, and the bitrate efficiencies of Beamr’s perceptually driven optimization. Over the years we have consistently validated that Beamr CABR technology creates optimized files that are perceptually identical, meaning they look the same to the human eye. While we have consistently demonstrated that the visual quality is indeed preserved, in this blog post we continue to explore how Beamr’s optimization lends itself to AI based workflows.
In our previous case studies, we looked at how the reduced bitrate, optimized videos, behave in Machine Learning (ML) tasks such as face detection and action recognition training. We showed that the results when using optimized AVC and HEVC encodes are stable, despite reducing file sizes significantly with an average reduction of 24% on the source files, and an amazing x3 decrease in size of the cropped AVC encoded files created by openCV.
Now we add codec modernization to the mix, which allows to reduce the sizes of the cropped encodes further. The AV1 encoded files are smaller by a factor of 4, while still providing very similar training and inference results, as shown by the maximal and average accuracy results obtained in the different experiments and presented in the following table:
Tested on AVC | Tested on optimized AV1 | |
Trained on AVC | 67.5% (53%) | 66.4% (53%) |
Trained on optimized AV1 | 66.4% (52%) | 64.8% (53.5%) |
Next we decided to ramp up the fun factor and play around with some cool AI applications. Using the open source Face Fusion project, we took 10 source AVC videos, an image containing our target face and proceeded to swap the faces in the source videos with our target person. Now, while this is a fun experiment in itself, imagine how much easier it becomes when the source videos are reduced by a factor of 4, with the results looking just the same.
Below is an example showing a frame from the source video, the target face image, and side by side comparison of the video with the replaced or fused face – when using the original AVC encode (on the left) or the AV1 optimized by Beamr (on the right), looking just as good:
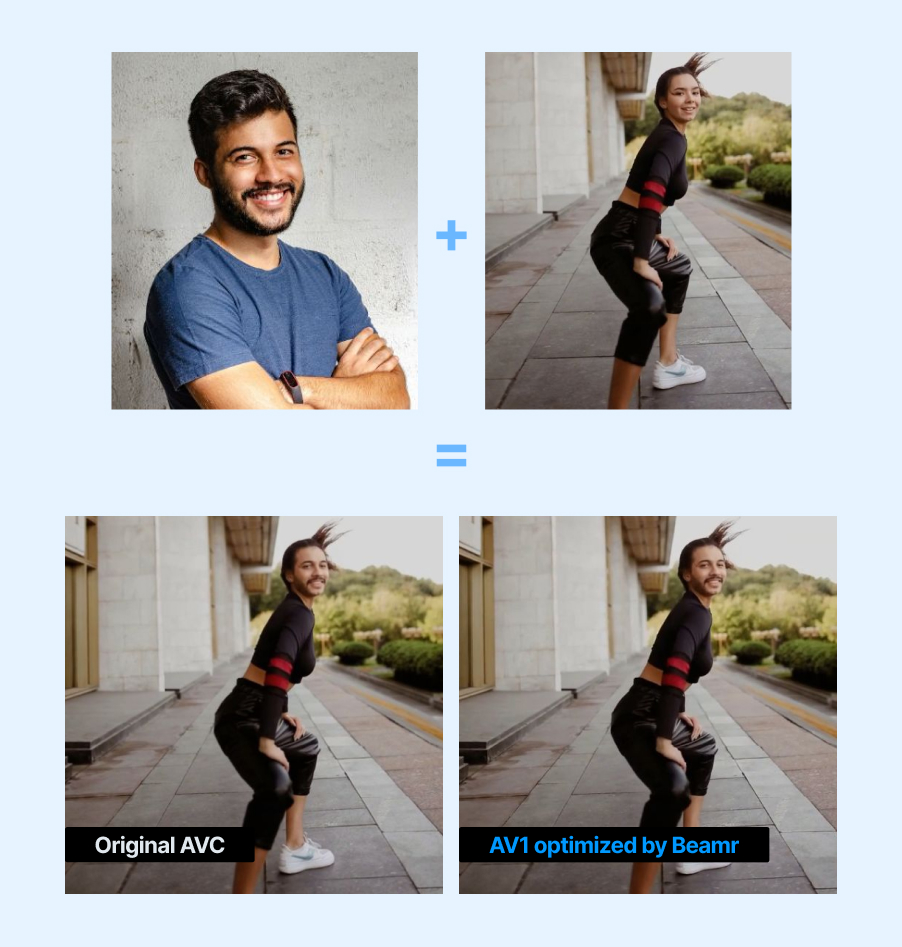
We are just starting to scratch the surface on how Beamr’s technology and offerings, including codec modernization to AV1, can help make AI workflows more efficient without compromising quality or accuracy. We are excited to be on this journey and will continue to explore and add on to the synergies between video optimization, video modernization and video AI solutions.