My Journey Through the Evolution of Video Processing: From Low-Quality Streaming to HD and 4K Becoming a Commodity, and Now the AI-Powered Video Revolution
Digital video has been my primary focus for the past three decades. I have built software codecs, designed ASICs, and now optimize GPU encoders with advanced GPU software.
My journey in video processing has been transformative, starting with low-resolution streaming, advancing into HD and 4K, as they shift from a rare event to an everyday expectation. And now, we stand at the next frontier—AI is redefining how we create, deliver, and experience video like never before.
My journey into this field began with the introduction of QuickTime 1.0 in 1991, when I was in my 20s. It looked to me like magic — a compressed movie playing smoothly on a single-speed CD-ROM (150 KB/s, 1.2 Mbps). At the time, I had no understanding of video encoding, but I was fascinated. At that moment I knew this is the field I wish to dive into.
Chapter 1: The Challenge of Streaming Video with Low-Resolution, Low-Quality Videos
The early days of streaming, in the mid 90s, were characterized by low-resolution video, low frame rates (12-15 fps), and low bitrates — 28.8 kbps, 33 kbps, or 56 kbps — two to three orders of magnitude 100x – 1000x lower bitrate than today’s standards. This was the reality of digital video in 1996 and the years followed.
By 1996, I was one of 4 co-founders of Emblaze – We developed a vector-based graphic tool called “Emblaze Creator” – think of it as Adobe Flash before Adobe Flash.
We soon realized we needed video support. We started by downloading videos in the background. Obviously, the longer the video was, the more time it took to download, which was frustrating to wait for. So we limited the videos to just 30 seconds.
Early solutions, like RealNetworks and VideoNet, required dedicated video servers — an expensive and complex infrastructure. It seemed to me like a very long and costly journey to streaming enablement.
Adding video to our offerings quickly was crucial for our company’s survival, so we persistently tackled this challenge. I remember the nights spent experimenting and exploring solutions, but all paths seemed to converge on the RealNetworks approach, which we couldn’t adopt in the short term.
We had to find a way to solve the challenge of streaming video efficiently for very low bandwidth. And while it was hard to stream files, you could slice them. So in 1997, I came up with an idea and worked with my team at Emblaze on the following solution:
- Take a video file and divide it into numbered slices.
- Create an index file with the order of the slices, and place it on a standard HTTP server.
- The player will read that index file and pull the segments from a web server in the order as in the index file.
Just to make it more real, here is the patent we submitted in 1998, and granted in 2002:
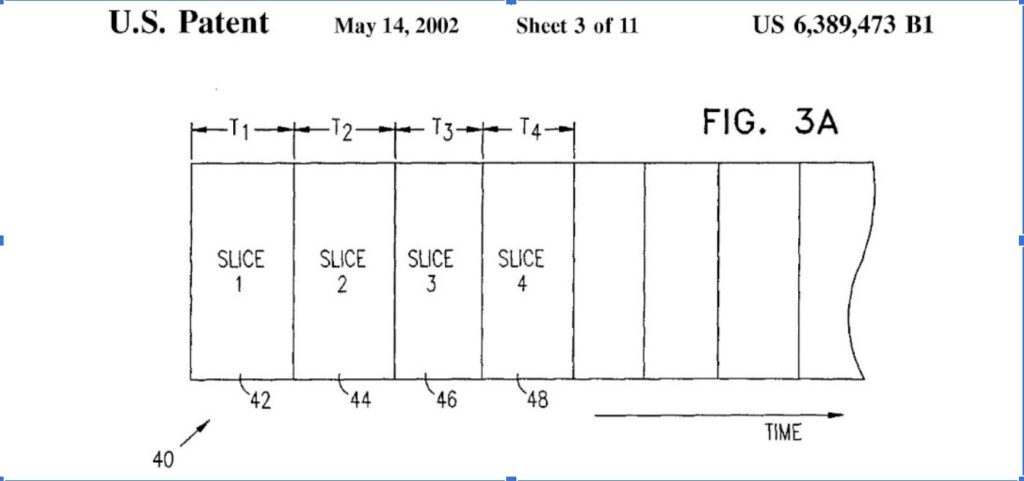
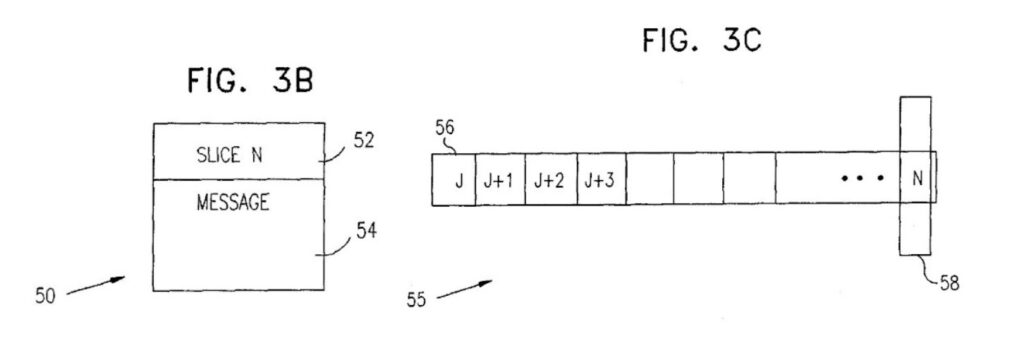
But that was not enough, why not create time synchronized slices, so the player will be able to pull the optimal chucks based on the specific bandwidth characteristics when playing the files?
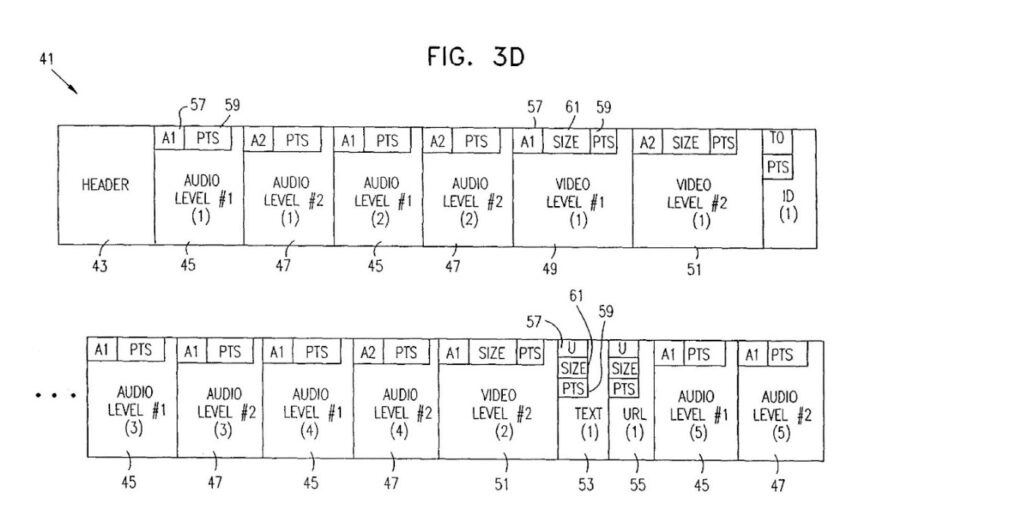
The player will read the index file from the server and choose a level to read, decide on a slice, and based on the bitrate move up and down the bitrate ladder.
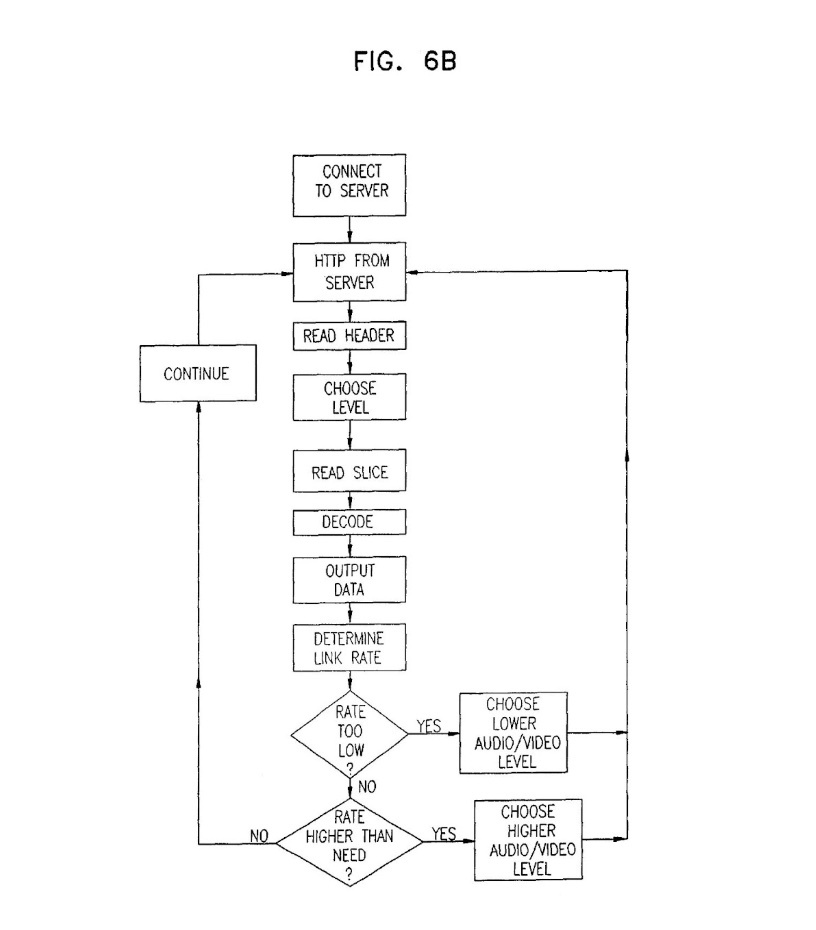
If that reminds you of HLS – then it was HLS many years before HLS was out.
We demonstrated this live with EarthLink at the Easter Egg Roll at the White House in 1998. Our systems were made of H.263 and then H.264 encoders, and a patented streaming protocol. We had a track with 10 Compaq workstations running 8 cameras that day.
When you build a streaming solution, you need a player. Without it, all that effort is meaningless. At Emblaze, we had a Java-based player that required no installation—a major advantage at the time.
Back then, mobile video was in its infancy, and we saw an opportunity. Feature phones simply couldn’t play video, but the Nokia Communicator 9110 could. It had everything—a grayscale screen, a 33MHz 32-bit CPU, and wireless data access—a powerhouse by late ‘90s standards.
In 1999, I demonstrated a software video decoder running on the Nokia 9110 to Samsung Mobile CEO. This was a game-changer—it proved that video streaming on mobile devices was possible. Samsung, being a leader in CDMA 2000, wanted to showcase this capability at the 2000 Olympics and needed working prototypes.
Samsung challenged us to build a mobile ASIC capable of decoding streaming video on just 100mW of power. We delivered. The solution was announced at the Olympics, and by 2001, it was in mass production.
This phone featured The Emblaze Multimedia Application Co-Processor, working alongside the baseband chip to enable seamless video playback over CDMA 2000 networks—a groundbreaking achievement at the time.
Chapter 2: HD Becomes the Standard, 4K HDR Becomes Common
HD television was introduced in the U.S. during the second half of the 90s, but it wasn’t until 2003 that satellite and cable providers really started broadcasting in HD.
I still remember 2003, staying at the Mandarin Oriental Hotel in NYC, where I had a 30-inch LCD screen with HD broadcasting. Standing close to the screen, taking in the crisp detail, was an eye-opening moment—the clarity, the colors, the sharpness. It was a huge leap forward from standard definition, and definitely better than DVDs.
But even then, it felt like just the beginning. HD was here, but it wasn’t everywhere yet. It took a few more years for Netflix to introduce streaming.
Beamr is Born
In early 2008, the startup I led, which focused on online backup, was acquired. By the end of the year, I found myself out of work. And so, I sent an email to Steve Jobs, pointing out that Time Machine’s performance was lacking, and that I believed I could help fix it. That email led to a meeting in Cupertino with the head of MobileMe—what we now know as iCloud.
That visit to Apple in early 2009 was fascinating. I learned that storing iPhone photos was becoming an enormous challenge. The sheer volume of images was straining Apple’s data centers, and they were running into power limitations just to keep up with demand.
With this realization, Beamr was born!
The question that intrigued us was: Can we make images smaller, while making sure they look exactly the same?
After about one year of research, we ended up founding Beamr instead of becoming a part of MobileMe. And the leader of this – the brains behind our technology that is here today.
During the first year of Beamr, we explored this idea. And we came out with our first product called JPEGmini, which does exactly that. This was achieved through the amazing innovation of our wonderful CTO, Tamar Shoham.
JPEGmini is a wonderful tool, and hundreds of thousands of content creators around the world use it.
After optimizing photos, we wanted to take on video compression. That’s when we developed our gravity defier—CABR, Content Adaptive BitRate technology. This quality-driven process can cut every high-quality video by 30% to 50% while preserving every frame’s visual integrity.
But our innovation comes with challenges:
- Lightning-fast encoding without CABR, but with CABR it is slower and can’t run live at 4Kp60.
- Running CABR is more expensive than non-CABR encoding.
In the year 2018, we came to the conclusion that we needed a hardware acceleration solution – to improve our density, our speed and the cost of processing.
We started by integrating with Intel GPUs, and it worked very well. We even demoed it at Intel Experience Day in 2019.
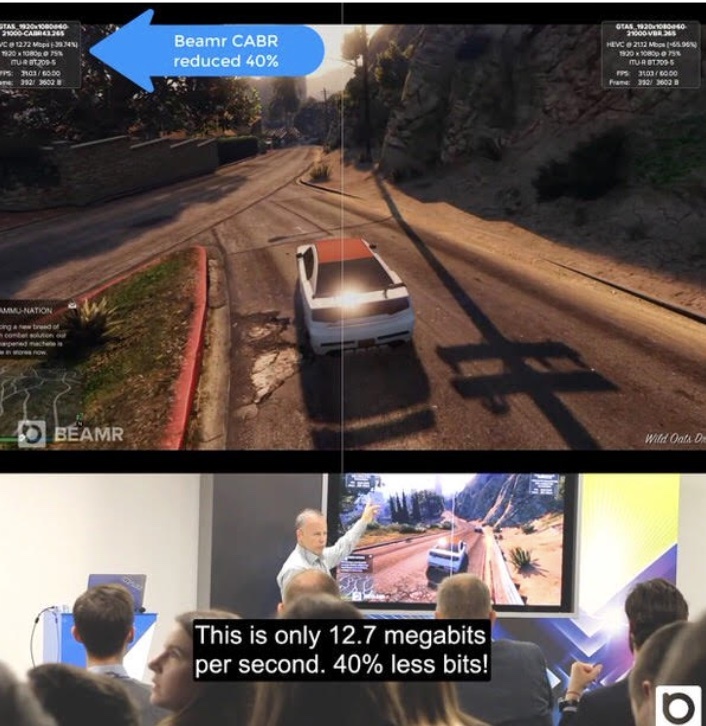
We had wonderful relationships with Intel and they had a good video encoding engine. We invested about two years of effort, and it did not materialize as an Intel GPU for the Data Center didn’t happen – a wasted opportunity.
Then, we thought of developing our own chip:
- Its power will be a function of CPU or GPU
- We will be able to put four 8Kp60 CABR chips on a single PCI card (for AC/HEVC and AV1).
- It will cost less than a GPU and have 3X density.
Here’s a slide that shows that we were serious. We also started a discussion about raising funds to build that chip using 12nm technology.
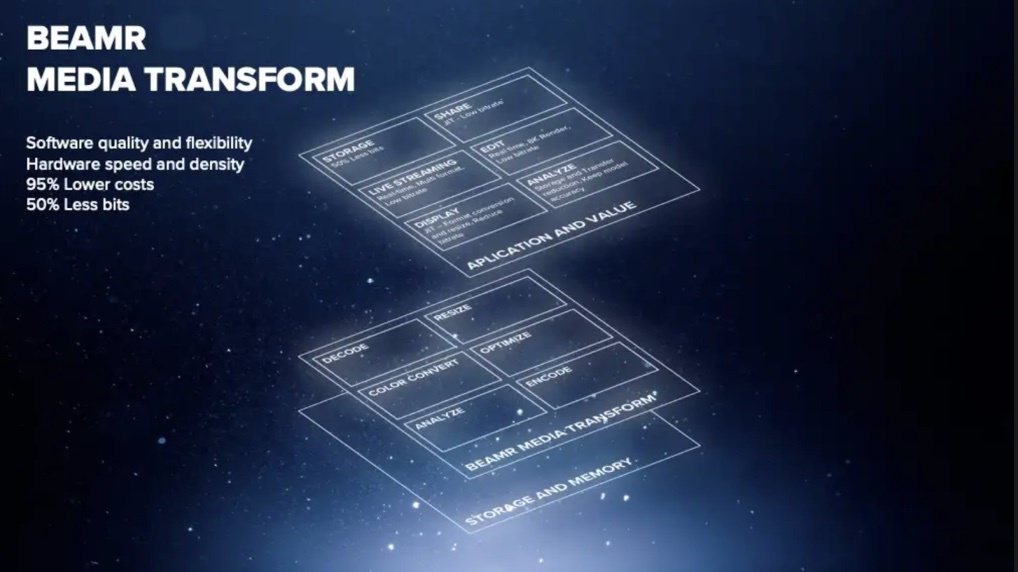
But then, we looked at our plan and wondered: does this chip support the needs of the future?
- How would you innovate on this platform?
- What if you would like to run smarter algorithms or a new version of CABR?
- Our design included programmable parts for customization. We even thought of adding GPU cores – but who is going to develop for it?
This was a key moment in 2020, when we understood that innovation is so fast that every silicon generation takes at least two years to build and that is too slow.
There is a scale that VPU solutions will be more efficient than GPU, but that cannot compete with the current pace of change. It may come that even the biggest social networks will abandon VPUs due to the need for AI and video to work together.
Chapter 3: GPUs and the Future of Video Processing
By 2021, NVIDIA invited us to bring CABR to GPUs. This was a three-year journey, requiring a complete rewrite of our technology for NVENC. NVIDIA fully supported us, integrating CABR into all encoding modes across AVC, HEVC, and AV1.
In May 2023, the first driver was out: NVENC SDK 12.1!
At the same time, Beamr went public on NASDAQ (under the ticker BMR), on the premise of a high-quality large-scale video encoding platform enabled on NVIDIA GPUs.
Since September 2024. Beamr CABR is running LIVE video optimization on NVIDIA GPUs at 4Kp60 across 3 codecs AVC, HEVC and AV1. It is 10X faster at 1/10 of the cost for AVC, and the ratio for HEVC is double – and you can double that again for AV1.
All of our challenges for bringing CABR to the masses are solved.
But the story doesn’t end here.
What we didn’t fully anticipate was how AI-driven innovation is transforming the way we interact with video, and the opportunities are even greater than we imagined, thanks to the shift to GPUs.
Let me give you a couple of examples:
In the last Olympics, I was watching windsurfing, and on-screen, I saw a real-time overlay showing the planned routes of each surfer, the wind speed and forward tactics, and the predictions on how they would converge at the finish line.
It was seamless, intuitive, and AI-driven—a perfect example of how AI enriches the viewing experience.
Or think about social media: AI plays a huge role in processing video behind the scenes. As videos are uploaded, VPUs (Video Processing Units) handle encoding, while AI algorithms simultaneously analyze content—deciding whether it’s appropriate, identifying trends, and determining who should see it.
But the processes used by many businesses are slow and inefficient. For every AI-powered video workflow, you need:
- Load the video.
- Decode it.
- Process it (either for AI analysis or encoding).
- Sync and converge the process.
Traditionally, these steps happened separately, often with significant latency.
But on a GPU?
- Single load, single decode, shared memory buffer.
- AI and video processing run in parallel.
- Everything is synced and optimized.
And just like that—you’re done. It’s faster, more efficient, and more cost-effective. This is the winning architecture for the future of AI and video at scale.
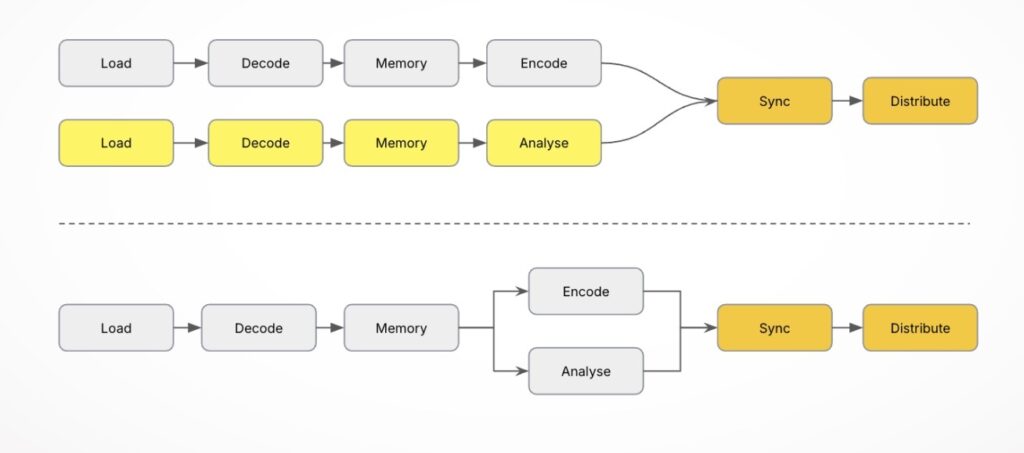
Want to learn more? Start a free trial with Beamr Cloud or Talk to us!